AI Guardrails: How Enterprises Can Safely Adapt to the Generative AI Wave
As AI adoption has exploded, companies are under more pressure than ever to figure out how to use this technology safely. We dig into key considerations and what questions companies need to be asking to prepare.
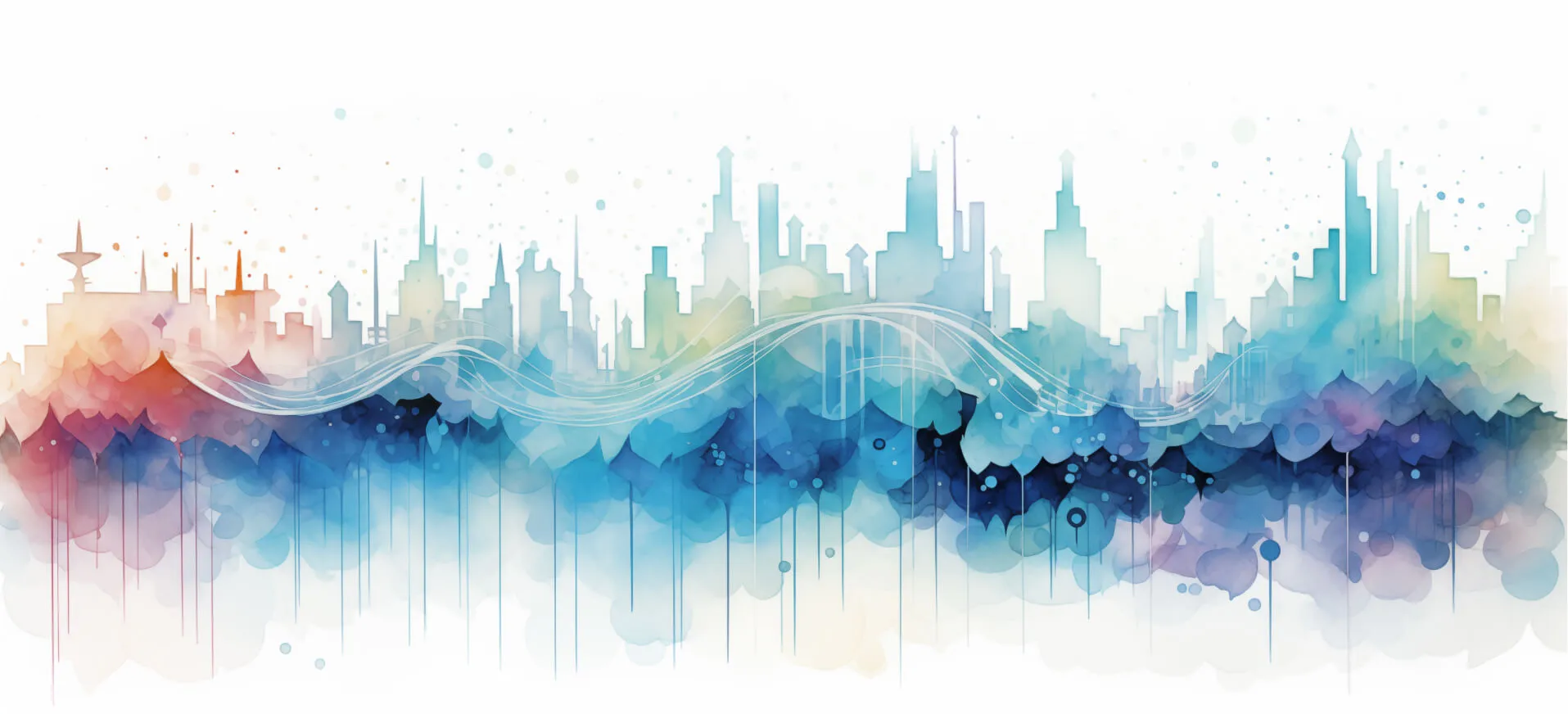
The abrupt and headline-making removal then reinstatement of OpenAI CEO Sam Altman—falling around the one-year anniversary of the release of OpenAI’s defining product, chatGPT—makes now a prime moment to reflect on the whirlwind past year of AI innovation.
In that time, we’ve witnessed a Cambrian explosion of AI tools, companies, and conversations, with generative AI projected to add $4.4 trillion to the global economy as automation boosts productivity.
Alongside this feverish activity, there is a deep need for guardrails built on legal, security, technological, and ethical considerations surrounding AI. As both new and established players enhance their AI offerings, companies with thoughtful guardrail strategies will be better positioned for long-term success than those flying fast, but blind.
At M13, we seek to understand the mechanisms, benefits, and risks of this technology, and to balance these perspectives in crafting our AI portfolio. Whether it’s protecting personal data, understanding how a model produced an answer, or ensuring compliance with laws and regulations, AI guardrails are a major consideration for us—and we see it as a key investment area today and in the future.
What will it take for enterprises to successfully adopt this new wave of generative AI technology? Below, we highlight some of the topics we’ve been thinking through—and the questions companies should be asking—as we navigate this bold era of AI.
The stakes are higher for enterprise
The use of AI comes with higher stakes for enterprises than for individuals, and for companies using this technology, proper guardrails will be crucial.
We like to compare AI adoption to the example of self-driving cars. Culturally, we ask for perfection from self-driving technology, and we can’t accept anything less, even though we do accept that human drivers will make mistakes. While we can accept that humans are fallible, there’s a feeling that technology can achieve perfect adherence to rules and guidelines.
Similarly, it makes us uncomfortable to accept a known failure rate from an AI agent—even if the failure rate is lower than what we typically see with human actors.
When a human employee makes a mistake or creates a bad outcome, that error is often seen as unique to that person and limited in impact radius. If an AI agent creates a bad outcome—mismanages a client’s money or recommends a poor treatment plan to a patient—it becomes a systemic issue, one that can implicate an entire company. The company as a whole is deemed responsible.
Consumers, regulatory bodies, and public opinion are less tolerant of AI failure than human failure.
Market map: AI enterprise requirements & guardrails
Below, we identify some of the startups and incumbents that enterprises are turning to in order to protect their data, ensure regulatory compliance, and better understand and optimize safe model outputs.
As investors, we are very interested in meeting companies focusing on end-to-end solutions to enable safe, flexible, and accurate usage of generative AI capabilities. We are also interested in how enterprises will solve concerns around IP infringement, especially in the creative world.
Are you building in this space, or do you want to be included in our market map? Reach out to anna@m13.co and morgan@m13.co.
Data security & protection
There is a core risk in managing the inputs to generative AI applications, and it’s important to consider the data security of confidential customer data. Already, many companies—including Microsoft, JPMorgan Chase & Co., and Apple—have created strict policies against sharing any customer data with outside genAI tools.
OpenAI is working to create data safeguards around the use of its tools. Still, it is likely that companies in highly regulated industries will need to employ data masking strategies by leveraging systems like Liminal.ai, Kobalt Labs, Credal, Presidio, and others, in order to use external models to generate AI outputs.
At M13, we’ve experimented with AI tools to create images for blog posts, take notes for certain meetings, and draft social and marketing copy—but our policy cautions against uploading sensitive proprietary information to genAI tools or using them to write content that requires deeper fact checking.
Questions companies should ask:
Levels of risk
Accuracy of output is an important consideration when it comes to generative AI tools, and soundly analyzing the level of risk that automation poses at large is crucial for developing a reasonable AI strategy. Within an industry or even within a type of task, different circumstances and processes will carry different levels of risk.
For example, take AI identification of images. Societally, we’re generally accepting of a photo app that fails to perfectly identify every picture of our friend in our camera roll—but we have low tolerance (and high penalties) for self-driving cars that misidentify pedestrians and potentially put them in harm’s way.
Below, we highlight some higher- and lower-risk processes to consider across industries. While this list is far from exhaustive, it does help illustrate how companies can begin approaching risk categorization:
Questions companies should ask:
Navigating the legal & regulatory landscape
Government and regulatory oversight of AI is heating up. In October, President Biden issued a landmark executive order calling for “safe, secure, and trustworthy artificial intelligence,” and last month the United States joined 30 other nations in agreeing to set guardrails for military AI in the first major international agreement of its kind. The EU also recently agreed on a draft for the AI Act, the first major piece of AI legislation.
In the prelude to Biden’s executive order, top AI companies—including Amazon, Google, Meta, Microsoft, and OpenAI—met and agreed to protect users through a series of cybersecurity and data transparency commitments. These voluntary commitments have so far focused on informing users about how data is used and keeping personal data secure, rather than commitments not to use data to train models or any warranties about the quality of outputs of AI models.
Regulatory concerns are not preventing the use of AI. A recent liminal.ai survey of workers across five heavily regulated industries (banking and finance, insurance, biotech and life sciences, manufacturing, and healthcare) found that two-thirds of respondents used generative AI tools on a weekly basis, and 90% agreed generative AI was at least somewhat valuable in helping with their work. Only 26% reported being fully prevented from using generative AI tools at work.
We expect to see significant movement in these results as more companies implement AI policies and roll out AI security tools.
Questions companies should ask:
Ownership & copyright
Ownership and copyright are crucial issues when it comes to genAI output, and we are a long way from resolution.
It’s certainly a topic on many creators’ minds: the tentative billion-dollar contract to end the months-long SAG-AFTRA strike includes provisions to “protect members from the threat of AI.” The deal that ended the Writers Guild of America strike prohibits studios from using generative AI to write or rewrite literary material or to require writers to use the technology.
Do the original authors of works used to train AI models have a stake in what those models generate? If so, how should that ownership stake be calculated? Do the prompt generators own the outputs that they generate, and how would such ownership be claimed?
One can imagine the US Copyright Office and USPTO being overrun with registrations, claims, and challenges to copyright as well as patent and trademark claims. An author might be satisfied seeing their name in a footnote or the acknowledgements section of a book or research paper without expecting compensation. But with AI, there’s a different calculus.
Going forward, we'll require an entirely new way of thinking about intellectual property in a world where the tools for creation are so widely available. This is an area that urgently needs regulatory or judicial guidance—if ownership of AI outputs is clouded, many people will simply stop using the tools.
Questions companies should ask:
Get in touch:
Are you building in the AI guardrails space? We want to talk. Reach out to our investing team by contacting anna@m13.co and morgan@m13.co.
The abrupt and headline-making removal then reinstatement of OpenAI CEO Sam Altman—falling around the one-year anniversary of the release of OpenAI’s defining product, chatGPT—makes now a prime moment to reflect on the whirlwind past year of AI innovation.
In that time, we’ve witnessed a Cambrian explosion of AI tools, companies, and conversations, with generative AI projected to add $4.4 trillion to the global economy as automation boosts productivity.
Alongside this feverish activity, there is a deep need for guardrails built on legal, security, technological, and ethical considerations surrounding AI. As both new and established players enhance their AI offerings, companies with thoughtful guardrail strategies will be better positioned for long-term success than those flying fast, but blind.
At M13, we seek to understand the mechanisms, benefits, and risks of this technology, and to balance these perspectives in crafting our AI portfolio. Whether it’s protecting personal data, understanding how a model produced an answer, or ensuring compliance with laws and regulations, AI guardrails are a major consideration for us—and we see it as a key investment area today and in the future.
What will it take for enterprises to successfully adopt this new wave of generative AI technology? Below, we highlight some of the topics we’ve been thinking through—and the questions companies should be asking—as we navigate this bold era of AI.
The stakes are higher for enterprise
The use of AI comes with higher stakes for enterprises than for individuals, and for companies using this technology, proper guardrails will be crucial.
We like to compare AI adoption to the example of self-driving cars. Culturally, we ask for perfection from self-driving technology, and we can’t accept anything less, even though we do accept that human drivers will make mistakes. While we can accept that humans are fallible, there’s a feeling that technology can achieve perfect adherence to rules and guidelines.
Similarly, it makes us uncomfortable to accept a known failure rate from an AI agent—even if the failure rate is lower than what we typically see with human actors.
When a human employee makes a mistake or creates a bad outcome, that error is often seen as unique to that person and limited in impact radius. If an AI agent creates a bad outcome—mismanages a client’s money or recommends a poor treatment plan to a patient—it becomes a systemic issue, one that can implicate an entire company. The company as a whole is deemed responsible.
Consumers, regulatory bodies, and public opinion are less tolerant of AI failure than human failure.
Market map: AI enterprise requirements & guardrails
Below, we identify some of the startups and incumbents that enterprises are turning to in order to protect their data, ensure regulatory compliance, and better understand and optimize safe model outputs.
As investors, we are very interested in meeting companies focusing on end-to-end solutions to enable safe, flexible, and accurate usage of generative AI capabilities. We are also interested in how enterprises will solve concerns around IP infringement, especially in the creative world.
Are you building in this space, or do you want to be included in our market map? Reach out to anna@m13.co and morgan@m13.co.
Data security & protection
There is a core risk in managing the inputs to generative AI applications, and it’s important to consider the data security of confidential customer data. Already, many companies—including Microsoft, JPMorgan Chase & Co., and Apple—have created strict policies against sharing any customer data with outside genAI tools.
OpenAI is working to create data safeguards around the use of its tools. Still, it is likely that companies in highly regulated industries will need to employ data masking strategies by leveraging systems like Liminal.ai, Kobalt Labs, Credal, Presidio, and others, in order to use external models to generate AI outputs.
At M13, we’ve experimented with AI tools to create images for blog posts, take notes for certain meetings, and draft social and marketing copy—but our policy cautions against uploading sensitive proprietary information to genAI tools or using them to write content that requires deeper fact checking.
Questions companies should ask:
Levels of risk
Accuracy of output is an important consideration when it comes to generative AI tools, and soundly analyzing the level of risk that automation poses at large is crucial for developing a reasonable AI strategy. Within an industry or even within a type of task, different circumstances and processes will carry different levels of risk.
For example, take AI identification of images. Societally, we’re generally accepting of a photo app that fails to perfectly identify every picture of our friend in our camera roll—but we have low tolerance (and high penalties) for self-driving cars that misidentify pedestrians and potentially put them in harm’s way.
Below, we highlight some higher- and lower-risk processes to consider across industries. While this list is far from exhaustive, it does help illustrate how companies can begin approaching risk categorization:
Questions companies should ask:
Navigating the legal & regulatory landscape
Government and regulatory oversight of AI is heating up. In October, President Biden issued a landmark executive order calling for “safe, secure, and trustworthy artificial intelligence,” and last month the United States joined 30 other nations in agreeing to set guardrails for military AI in the first major international agreement of its kind. The EU also recently agreed on a draft for the AI Act, the first major piece of AI legislation.
In the prelude to Biden’s executive order, top AI companies—including Amazon, Google, Meta, Microsoft, and OpenAI—met and agreed to protect users through a series of cybersecurity and data transparency commitments. These voluntary commitments have so far focused on informing users about how data is used and keeping personal data secure, rather than commitments not to use data to train models or any warranties about the quality of outputs of AI models.
Regulatory concerns are not preventing the use of AI. A recent liminal.ai survey of workers across five heavily regulated industries (banking and finance, insurance, biotech and life sciences, manufacturing, and healthcare) found that two-thirds of respondents used generative AI tools on a weekly basis, and 90% agreed generative AI was at least somewhat valuable in helping with their work. Only 26% reported being fully prevented from using generative AI tools at work.
We expect to see significant movement in these results as more companies implement AI policies and roll out AI security tools.
Questions companies should ask:
Ownership & copyright
Ownership and copyright are crucial issues when it comes to genAI output, and we are a long way from resolution.
It’s certainly a topic on many creators’ minds: the tentative billion-dollar contract to end the months-long SAG-AFTRA strike includes provisions to “protect members from the threat of AI.” The deal that ended the Writers Guild of America strike prohibits studios from using generative AI to write or rewrite literary material or to require writers to use the technology.
Do the original authors of works used to train AI models have a stake in what those models generate? If so, how should that ownership stake be calculated? Do the prompt generators own the outputs that they generate, and how would such ownership be claimed?
One can imagine the US Copyright Office and USPTO being overrun with registrations, claims, and challenges to copyright as well as patent and trademark claims. An author might be satisfied seeing their name in a footnote or the acknowledgements section of a book or research paper without expecting compensation. But with AI, there’s a different calculus.
Going forward, we'll require an entirely new way of thinking about intellectual property in a world where the tools for creation are so widely available. This is an area that urgently needs regulatory or judicial guidance—if ownership of AI outputs is clouded, many people will simply stop using the tools.
Questions companies should ask:
Get in touch:
Are you building in the AI guardrails space? We want to talk. Reach out to our investing team by contacting anna@m13.co and morgan@m13.co.
Read more
The views expressed here are those of the individual M13 personnel quoted and are not the views of M13 Holdings Company, LLC (“M13”) or its affiliates. This content is for general informational purposes only and does not and is not intended to constitute legal, business, investment, tax or other advice. You should consult your own advisers as to those matters and should not act or refrain from acting on the basis of this content. This content is not directed to any investors or potential investors, is not an offer or solicitation and may not be used or relied upon in connection with any offer or solicitation with respect to any current or future M13 investment partnership. Past performance is not indicative of future results. Unless otherwise noted, this content is intended to be current only as of the date indicated. Any projections, estimates, forecasts, targets, prospects, and/or opinions expressed in these materials are subject to change without notice and may differ or be contrary to opinions expressed by others. Any investments or portfolio companies mentioned, referred to, or described are not representative of all investments in funds managed by M13, and there can be no assurance that the investments will be profitable or that other investments made in the future will have similar characteristics or results. A list of investments made by funds managed by M13 is available at m13.co/portfolio.